The programmatic advertising industry is experiencing a rapid blooming time: new companies are entering the market, technologies are snowballing, and powerful transformations are in the air. All of this is incredibly complicating the advertising ecosystems, creating conditions for increased fraud and waste.
This is where trending solutions that use machine learning (ML) and artificial intelligence (AI) models to predict rates and adjust bidding strategies in real time come into play, to everyone’s delight. However, instead of sighs of relief, a new drama unfolds: it turns out that AI-driven programmatic solutions do not solve all problems. Moreover, they cause new challenges and issues.
This article will analyze the peculiarities of using artificial intelligence models in programmatic advertising, especially within predictive bidding. Since AI is widely adopted and highly idealized, we will look behind the scenes of business goals for transparency and clarity and touch upon the development process.
Artificial Intelligence and Machine Learning for Optimization’s Sake
To begin the discussion, let’s answer the question of AI’s role in programmatic: it is significant and is expected to increase over time. Nevertheless, the same can be said about any field within information technologies, so let’s deal with the essence, not the wrapper of pretty words.
At a fundamental level, AI models in programmatic advertising are designed to predict the probability of achieving the expected result during trading. Accordingly, if the expected outcome is achievable, resources should be allocated to attain it. If it is not feasible, resources should be saved for future auctions. This is how it works. However, hundreds of devils are in the details, and they constantly shuffle them, trying to complicate the work of engineers and developers.
Implementing custom AI models presents significant challenges. Here, we will discuss these difficulties to give an accurate picture of the engineering world and the role of people who keep their finger on the pulse of automation.
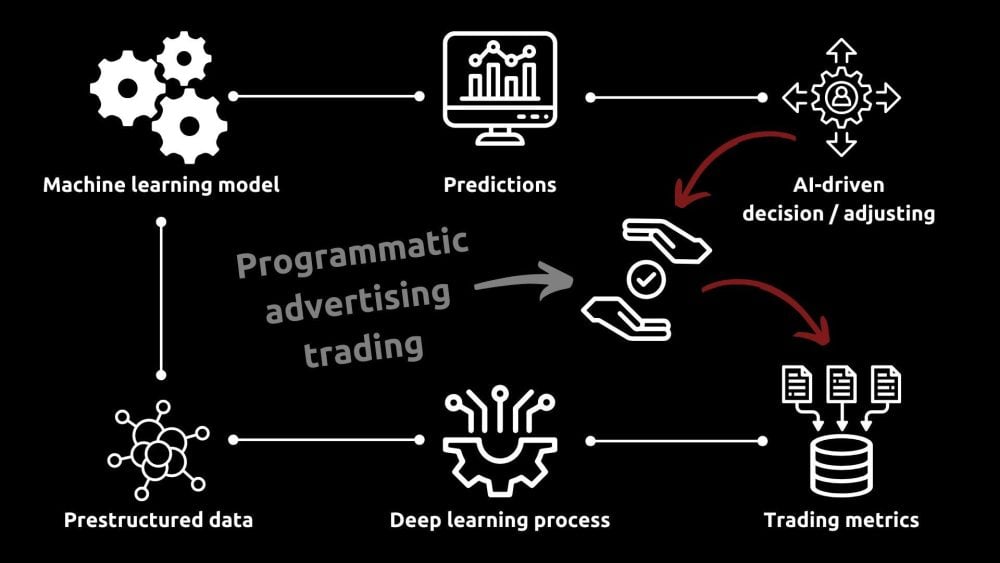
Programmatic advertising operates in an ecosystem of SSPs, DSPs, ad exchanges, and other trading participants. All these parties are technically complex applications deployed on servers. In this case, a custom AI model is a software solution developed, launched, and maintained by a specific participant of the advertising chain on its side. There are several essential points to understand the tension here.
- An AI model is typically deployed to optimize bidding with a specific partner, so stable and significant trading interactions and historical engagement are fundamental.
- The more significant the partner is for your portfolio, the better because you must train the model on large data sets for successful automation and algorithm improvement.
- The most effective approach to optimizing trading with predictive AI is implementing models that focus on improving one specific metric instead of multiple metrics simultaneously.
Fundamentally, for effective trading optimization by several metrics for multiple partners, you need to deploy a whole set of custom AI solutions that can be united by one guiding system principle. We will discuss this further.
Best Practices in Implementing AI Solutions for Boosting Performance in Programmatic
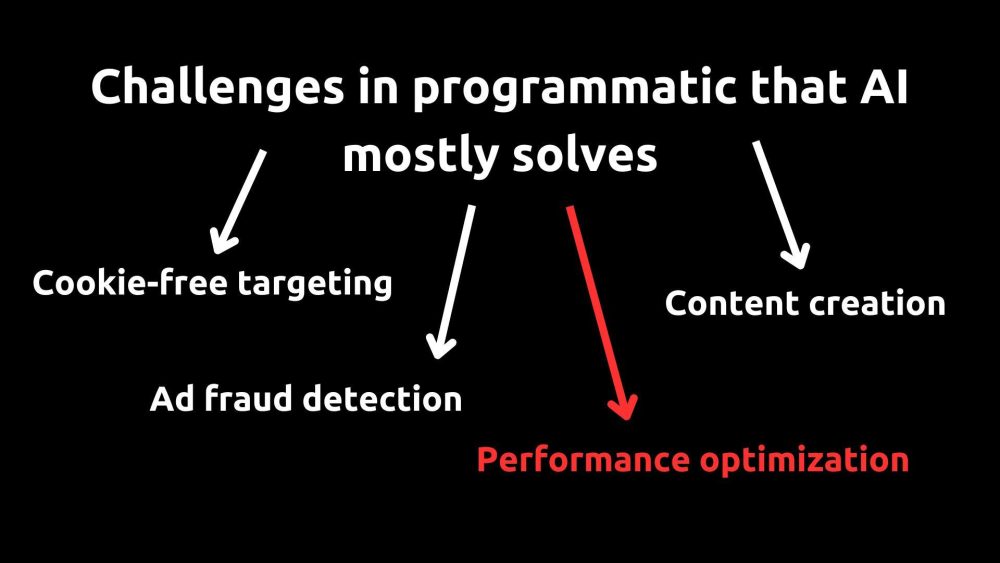
The role of artificial intelligence and machine learning is growing proportionally and predictably with the growing complexity of the digital advertising industry.
For illustration, Google’s sincere intention to ban third-party cookies by default for its Chrome browser is one of the catalysts for developing automated solutions that should partially or wholly compensate for the loss of targeting efficiency. However, in the programmatic industry, machine learning features and AI algorithms have already become routine elements in one form or another. The growth of automation is only a matter of time.
Below, we present practices assuming that traditional development approaches are well understood. These insights were mined from passionate ad tech people’s stern and stealthy work.
Invest in improving and controlling the data quality used to train and improve the AI model for predictive bidding.
This is the first thing that needs to be addressed by your development department or the team involved. Programmatic advertising is an area where discrepancies in the calculation of specific metrics often arise due to the presence of numerous bidders, imperfections in the methods and approaches used, and other reasons. Other reasons include the intentional distortion or misrepresentation of metrics data recorded by the system to gain an unethical advantage in auctions.
In the long history of ad tech spoofing, examples of data distortion include click injections, falsified location data, duplicated identifiers, and many more. Unfortunately, such types of fraud in programmatic can go unnoticed for many years.
Here is the first conclusion: a good AI model can be backed up with false data, forcing it to adjust the bidding not in favor of your business. This point should be eliminated by creating a multi-layered process of verifying AI-driven decisions by isolating a part of traffic and conducting various automated tests.
Link updates of automated models to local upgrades of the programmatic advertising infrastructure.
AI models are sensitive to server architecture. Suppose your partner suddenly upgrades its advertising platform to a new version and changes the internal logic behind the platform. In that case, it will definitely affect how the predictive analytics model perceives the data. Rarely are the changes so insignificant that they don’t affect the performance. Most often, even minor updates require analyzing and adjusting the analysis algorithms of the developed models. This is not to mention cases when partners switch the metrics calculation and transmission principle without warning the parties.
If a model was trained on one data set, but circumstances have changed, it is worth retraining it to reflect the shift.
Let’s overview an example from our practice. We constantly monitor updates by major market players, whose data we use to create custom client algorithms: specific adopted metrics may have changed after the update, affecting the algorithmic performance.
Supervise the changes in your partners’ optimization approaches. Automated analysis of the algorithms’ behavior is essential.
This is also an interesting point, as your advertising partners may change the focus to metrics they hadn’t previously observed. This will likely lead to difficulties in analyzing information and errors in decision-making that the developed predictive bidding model will face. Indeed, the ability to track internal changes in partners’ approaches is a difficult skill, but nothing is impossible if you prioritize efficiency.
Signs of shifts in the company’s optimization focus include changes in key technical executives, loss of funding or, vice versa, receiving funding, changes in the subscription model, such as Unity’s controversial Runtime Fee, and much more.
The conclusion is that the optimization is only effective under the current trading conditions with a particular partner, which can change and affect the performance. You can predict changes by analyzing public data about your partners. Still, automated models for analyzing and patterning partner trading behavior are far more critical insofar as this is possible on your part without crossing the line of ethics.
Follow the rule that predictive bidding with ML and AI solutions is a multi-player game that can lead to conflicts.
That’s why one of the best optimization rules is to have a break in the logic of applying the rules. If, during the break, the system “realizes” that the target metrics have improved, the further optimization approach should be reconsidered. For instance, some traffic continues to be optimized, some do not, and the results are compared to adjust further actions.
Predictive Bidding for Rapid Website Monetization
One of our clients, a large US-based SSP platform that uses TeqBlaze white-label solution to manage its business, contacted us with a problem. The client noticed that new publishers were complaining about low profits, and this problem could not be solved by following the best optimization practices deployed. So, the client asked us to find the problem and solve it technically in a unique manner.
After conducting a preliminary analysis of the platform, we have confirmed that trading conditions were almost the same for newcomers and major supply partners. Following this conclusion, we identified the issue within publishers’ inventory settings. Their win rate was much lower, so the main suspicion immediately fell on an incorrectly defined bid floor setting. To challenge our theory, we ran tests that proved that the inflexible bid floor led to a low win rate at the auctions.
Based on this, we added an ML algorithm to the client’s platform to help choose the optimal bid floor for partners. We’ve used the isolated part of the traffic for seamless development.
After deploying the designed and well-tested machine learning algorithm, the client achieved about a 2-3x improvement in win rate and a 30-35% increase in profits. Since this is ML, we constantly monitor the performance of the client’s platform and work on improving algorithms based on its data.
Automation’s Evolution Matters
Times are changing fast, but technology is evolving three times faster. The only thing that remains unchanged is people’s desire to have an impact, stay ahead, and predict the situation. Instead of promises of everything at once, sincerity and gradual partnership growth are gaining value. While AI offers tremendous potential to streamline bidding processes, the optimization journey continues to be as complex as rewarding. Behind the scenes of magic automation, honest people are still dedicated to their work and focused on strategic improvements for businesses in the face of great suspense.
We invite you to contact us if you want to optimize your programmatic advertising infrastructure with intelligent technologies.
Contact Teqblaze