The landscape of visual creation has undergone a seismic shift in recent years, as artificial intelligence (AI) has emerged as a powerful new tool in the artist’s arsenal. What started as experimental research projects has blossomed into sophisticated platforms capable of generating stunning, high-quality images from simple text descriptions (GenAI). This technological revolution has democratized visual creation, allowing anyone with an idea to bring their vision to life through AI.
AI Image Generation
At its core, ai-powered image generation represents a fascinating intersection of computer science, art, and human creativity. The technology has evolved rapidly from producing crude, abstract outputs to creating photorealistic images nearly indistinguishable from human-created work. But how does this seemingly magical process work?
The foundation of modern AI image generation lies in what researchers call diffusion models. These sophisticated neural networks learn by studying millions of images, gradually understanding how to construct visual elements from pure noise. The process mirrors how an artist might start with a blank canvas and progressively build up a complete image, but at a mathematical level that operates on pixel patterns and learned visual concept representations.
During training, these models learn by working backward. They start with clear images and gradually add random noise until the pictures become unrecognizable. This process teaches them to understand the relationship between noise and meaningful visual information. When generating new images, they reverse this process, starting with random noise and gradually refining it into coherent pictures guided by text descriptions.
AI Image Generation Platforms
The current generation of AI image generators has achieved remarkable capabilities. They can create everything from photorealistic portraits to fantastical landscapes, from product mockups to abstract art. The technology has found applications across numerous fields, from advertising and product design to conceptual art and entertainment.
Several prominent platforms are leading the charge in this revolution, each with its own strengths and characteristics.
- Midjourney has gained recognition for its artistic flair, consistently producing visually striking images that lean toward the creative and imaginative. Its results often carry a distinctive aesthetic that has become recognizable to those familiar with AI art.
- DALL-E, created by OpenAI, approaches image generation with a more versatile toolset. It excels at understanding complex prompts and producing photorealistic images, making it particularly useful for commercial applications and conceptual visualization. The platform has demonstrated an impressive ability to understand and execute nuanced requests, though, like all current systems, it still has its limitations.
- Stable Diffusion has taken a different path by embracing open-source development. This has led to a flourishing ecosystem of tools and interfaces built around its core technology. Users can run the system locally on their hardware, customize its behavior, and even fine-tune it for specific use cases. This openness has made it a favorite among technical users and those looking to push the boundaries of what’s possible with AI image generation.
Getting started with AI image generation requires understanding not just the tools but also the art of prompting—the craft of communicating effectively with these AI systems. Success often lies in finding the right balance between being specific enough to guide the AI toward your vision while leaving enough room for the system to apply its learned understanding of aesthetics and composition.
Prompting AI Image Creators
While text and image generation use natural language prompts, image prompting requires a fundamentally different approach. With text generation prompting, we can use conversational language and rely on the AI’s understanding of context and flow.
Image generation through AI presents a unique challenge regarding iteration, fundamentally different from text generation, where you can refine and build upon previous outputs. Each generated image represents a fresh interpretation of the prompt from random noise rather than modifying a previous result. Image generation requires more precise, descriptive language that builds a complete visual scene. Think of it like the difference between telling a story and painting a picture with words – every detail must be explicitly stated each time because the AI can’t infer visual context or iterate the way it can with text.
When you say, Make the trees taller or Add more blue, the AI doesn’t modify the last image – instead, it starts over with a new generation, interpreting your entire prompt anew. This is why seemingly small prompt changes can sometimes produce dramatically different results and why getting from almost right to perfect can be frustrating.
The AI isn’t seeing and tweaking your previous image (except in specific cases like img2img or inpainting); it’s creating an entirely new image from scratch based on its training data and prompt. This means that achieving consistent results often requires very precise prompt engineering, and each generation is essentially a fresh attempt rather than a true iteration. Understanding this fundamental aspect of AI image generation helps explain why fine-tuning an image often feels less like sculpting and more like repeatedly rolling dice with slightly different weights.
AI Image Prompt Example
Let’s build a prompt step-by-step, starting with a basic concept of a magical forest scene. We’ll see how each layer of detail transforms the output. I’m going to use Hugging Face’s Stable Diffusion space for this demonstration.
- Basic Subject: Start with the core subject or scene. This forms the foundation of your image.
magical forest clearing at night
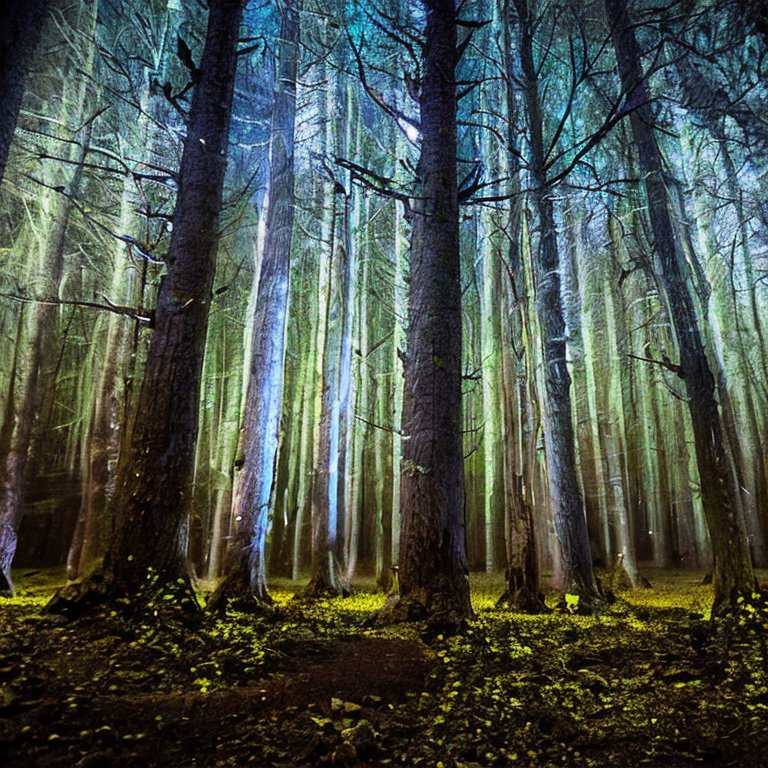
- Lighting and Atmosphere: Add the primary lighting and atmospheric conditions. This dramatically affects the mood and depth of the image.
magical forest clearing at night, illuminated by bioluminescent mushrooms and floating orbs of blue light, misty atmosphere, moonbeams filtering through the canopy
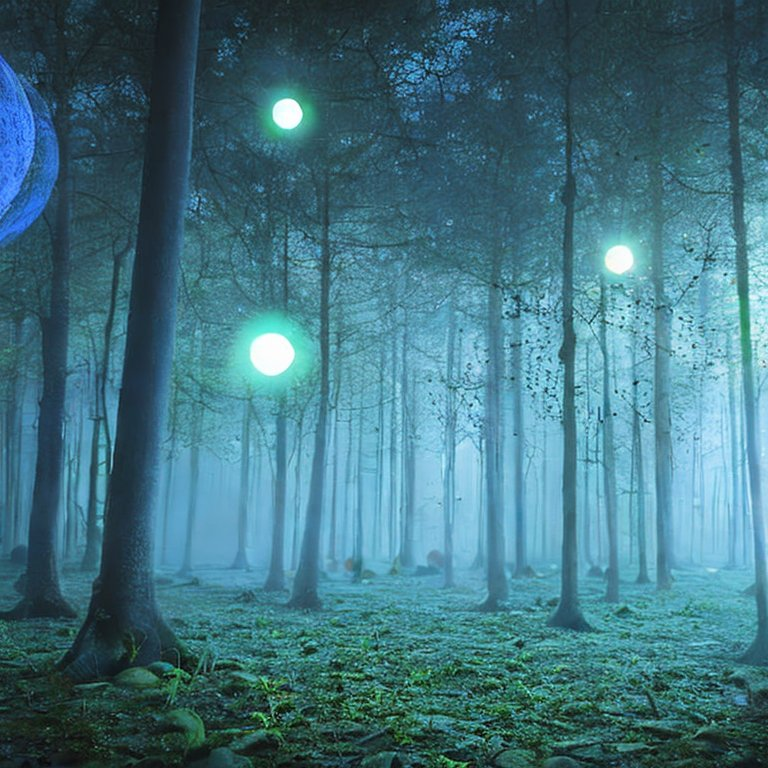
- Composition and Perspective: Define how the scene is framed and viewed. This helps create a more intentional, artistic result.
magical forest clearing at night, illuminated by bioluminescent mushrooms and floating orbs of blue light, misty atmosphere, moonbeams filtering through the canopy, dramatic wide-angle shot from ground level, foreground elements frame the scene
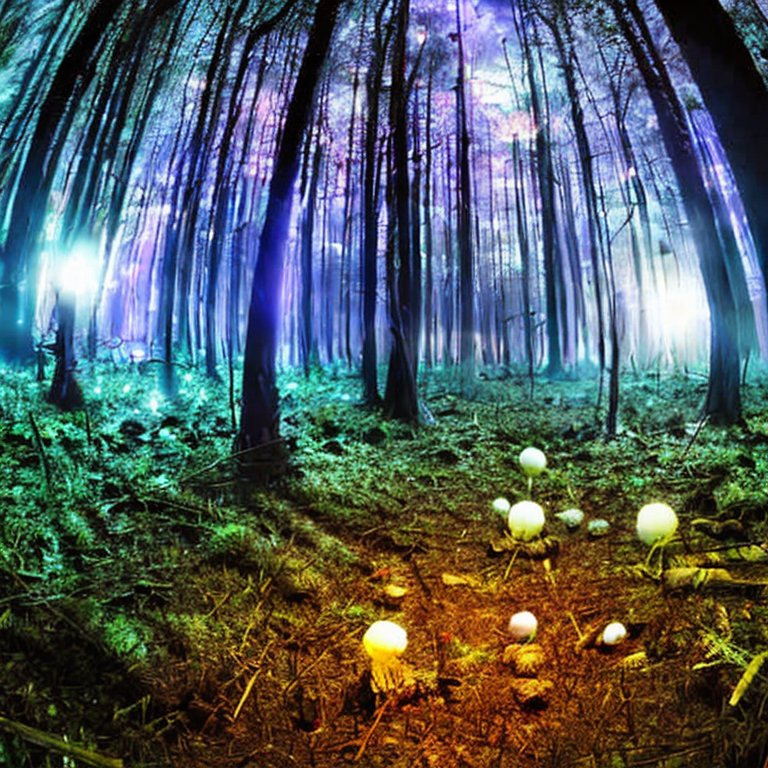
- Material and Texture Details: Specify the physical qualities of key elements. This adds richness and tactile quality to the image.
magical forest clearing at night, illuminated by bioluminescent mushrooms and floating orbs of blue light, misty atmosphere, moonbeams filtering through the canopy, dramatic wide-angle shot from ground level, foreground elements frame the scene, ancient gnarled tree roots covered in phosphorescent moss, dewy cobwebs catching the light
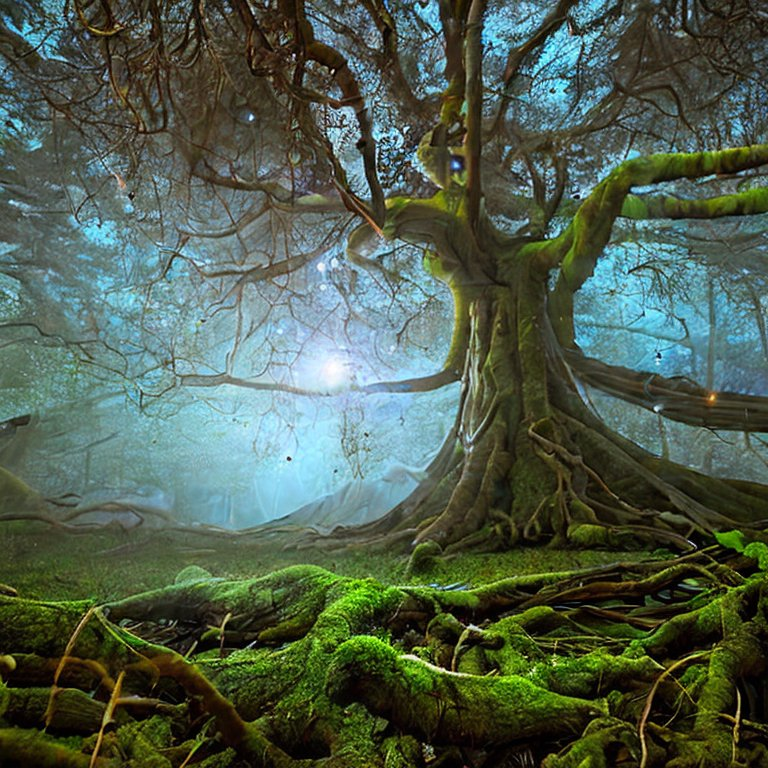
- Color Palette: Define the specific colors and their relationships. This creates visual cohesion.
magical forest clearing at night, illuminated by bioluminescent mushrooms and floating orbs of blue light, misty atmosphere, moonbeams filtering through the canopy, dramatic wide-angle shot from ground level, foreground elements frame the scene, ancient gnarled tree roots covered in phosphorescent moss, dewy cobwebs catching the light, rich deep blues and teals with accents of glowing cyan and purple
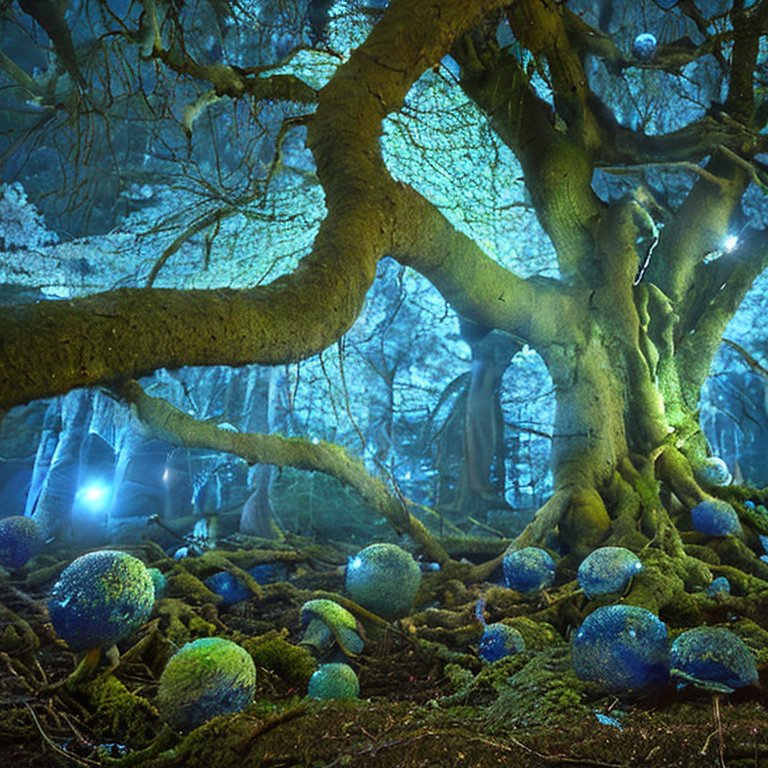
- Artistic Style: Specify the rendering style and artistic influence. This shapes the overall aesthetic.
magical forest clearing at night, illuminated by bioluminescent mushrooms and floating orbs of blue light, misty atmosphere, moonbeams filtering through the canopy, dramatic wide-angle shot from ground level, foreground elements frame the scene, ancient gnarled tree roots covered in phosphorescent moss, dewy cobwebs catching the light, rich deep blues and teals with accents of glowing cyan and purple, rendered in the style of Studio Ghibli meets digital concept art, hyperdetailed
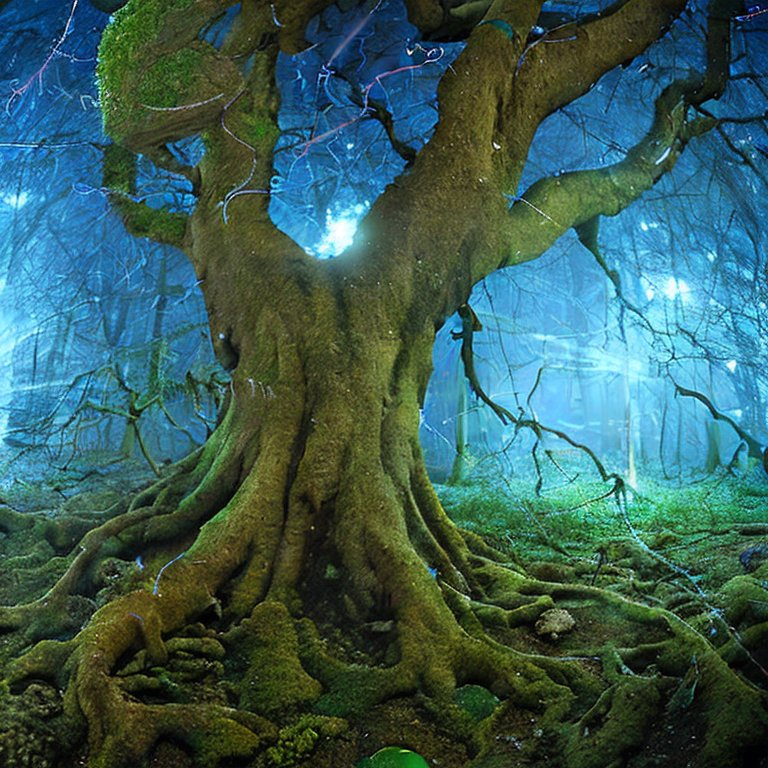
- Technical Specifications: Add parameters that affect the technical quality of the output.
magical forest clearing at night, illuminated by bioluminescent mushrooms and floating orbs of blue light, misty atmosphere, moonbeams filtering through the canopy, dramatic wide-angle shot from ground level, foreground elements frame the scene, ancient gnarled tree roots covered in phosphorescent moss, dewy cobwebs catching the light, rich deep blues and teals with accents of glowing cyan and purple, rendered in the style of Studio Ghibli meets digital concept art, hyperdetailed, 8K resolution, cinematic aspect ratio, volumetric lighting
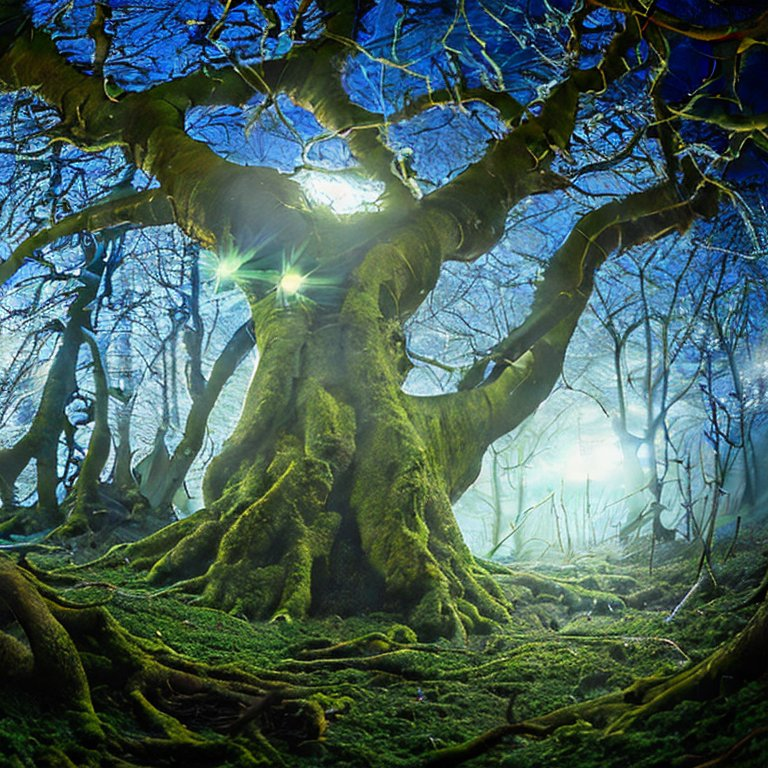
- Enhancement Keywords: Add specific terms many AI models recognize as quality boosters.
magical forest clearing at night, illuminated by bioluminescent mushrooms and floating orbs of blue light, misty atmosphere, moonbeams filtering through the canopy, dramatic wide-angle shot from ground level, foreground elements frame the scene, ancient gnarled tree roots covered in phosphorescent moss, dewy cobwebs catching the light, rich deep blues and teals with accents of glowing cyan and purple, rendered in the style of Studio Ghibli meets digital concept art, hyperdetailed, 8K resolution, cinematic aspect ratio, volumetric lighting, award-winning, masterpiece, photorealistic rendering, professional photography, trending on artstation
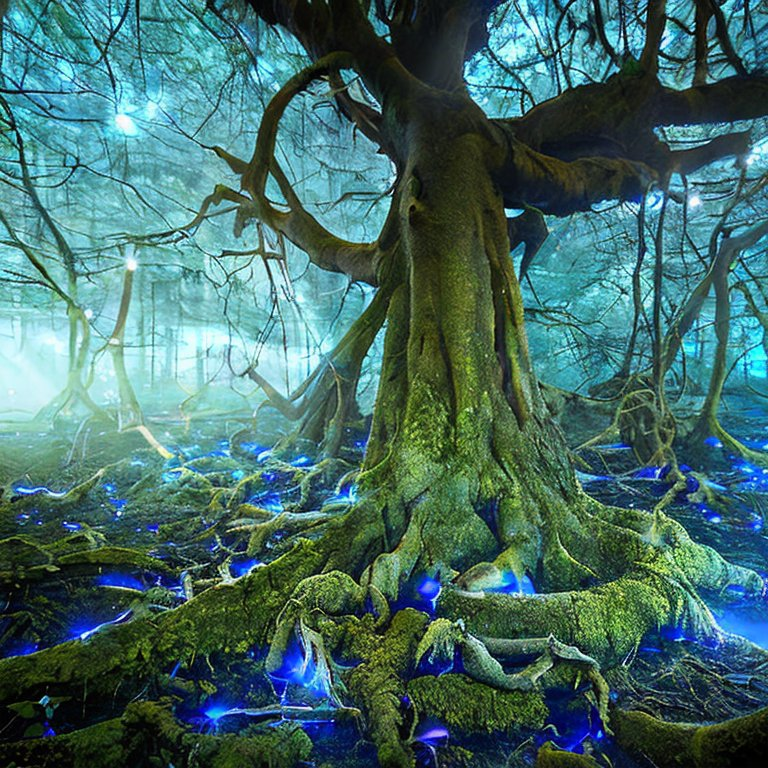
- Negative Prompt: Finally, specify what you don’t want to see. This helps avoid common AI artifacts or unwanted elements. Note that different platforms handle negative prompts differently – some use separate fields, others use specific syntax.
[Positive prompt as above]
Negative prompt: blurry, poor composition, washed out colors, oversaturated, lens flare, chromatic aberration, poor lighting, deep fried, poor shadows, blown out highlights, web watermarks, text, signatures, ugly, disfigured, deformed, plastic looking
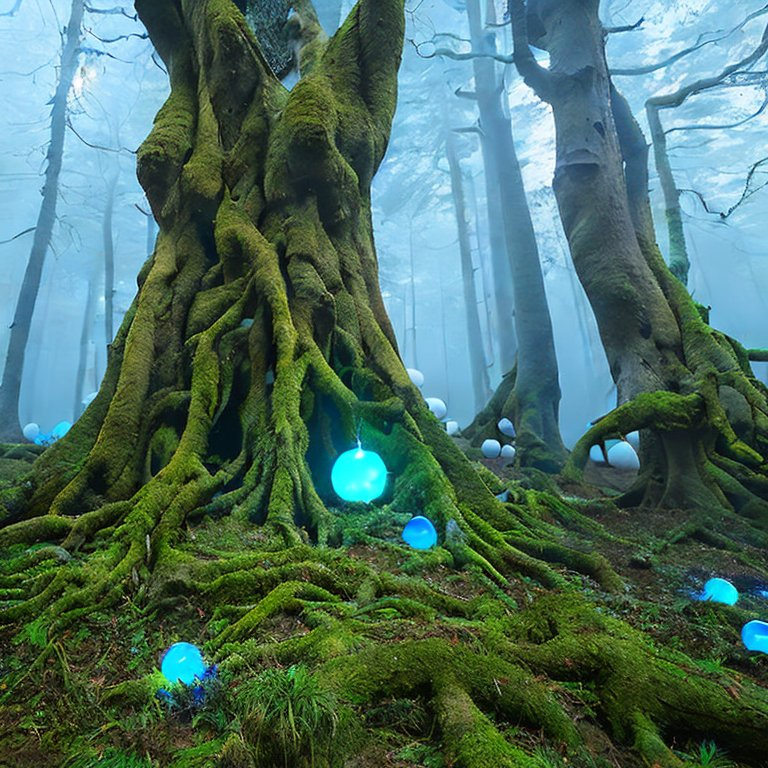
When using these prompts, remember:
- Different AI models respond differently to prompts – what works perfectly in Midjourney might need adjustment for Stable Diffusion.
- The order of elements can matter – the most important details should come earlier in the prompt.
- Use commas to separate distinct concepts.
- Avoid contradictory descriptions.
- Be specific about important details, but leave room for the AI to interpret artistic elements.
Understanding the underlying parameters of image generation can significantly improve results. This includes sampling methods, which control how the AI refines its output; seed numbers, which allow for reproducibility; and negative prompts, which help define what the system should avoid. While these technical details aren’t necessary for basic use, they become valuable tools for achieving precise, consistent results.
The Future and Ethical Considerations
The potential of AI image generation extends far beyond simple picture creation. These tools are increasingly integrated into creative workflows, serving as collaborative partners in the creative process. Artists and designers use them to rapidly prototype ideas, explore variations, and push the boundaries of their imagination.
However, this technology also raises important ethical considerations. Questions about copyright, artistic attribution, and the impact on human artists remain at the forefront of discussions in the creative community. As these tools become more powerful and widespread, developing frameworks for their responsible use becomes increasingly important.
The future of AI image generation promises even more exciting developments. Researchers are improving fine control over generated images, better understanding of physical constraints, and more sophisticated handling of text and complex scenes. The technology continues to evolve rapidly, with new capabilities and improvements emerging regularly.
The journey begins with experimentation for those looking to explore AI image generation. Start with simple prompts and gradually build up to more complex requests as you understand how the systems interpret and respond to different instructions. Join community forums, study successful examples, and don’t be afraid to push the boundaries of what’s possible.
As we look to the future, AI image generation stands as a testament to artificial intelligence’s potential to augment and enhance human creativity rather than replace it. The technology continues to evolve, offering ever more sophisticated tools for visual creation while raising important questions about the nature of creativity and authorship in an AI-assisted world.
The revolution in AI image generation is just beginning. As these tools become more sophisticated and accessible, they will continue to reshape how we approach visual creation, opening new possibilities for artistic expression and commercial application. The key to success lies in understanding both these systems’ capabilities and limitations and learning to work with them as collaborative tools in the creative process.